🧠 ‘AI Alignment’ isn’t a problem — it’s a myth.
Come with me on a journey through ordered and unordered systems
November involved lots of carbs, gratitude, and Untangled:
📚I launched the first ever ‘AI Reading List’
🗞️ I Untangled the News, synthesizing the best academic papers and news articles I read over the month.
Want more of this piped into your inbox? You know what to do.
Now, on to the show!
I’ve written about the false promise of emergence, and why AI doomers are wrong to fear that we might build a rogue AI that is so powerful we can’t control it. But there is another, much more thoughtful group of people working on ‘the alignment problem,’ who believe that if we try hard enough, we can align AI with our needs and wants. They too are making a mistake in their assumptions — albeit in a less glaring way. See, I don’t think AI alignment is a problem to solve — I think it’s a myth. Let’s dig in.
‘The alignment problem’ was popularized by Brian Christian in a great book by the same name. Christian explains that human values, wants, and needs cannot easily align with the outputs of AI. For example, Christian asks that we critically consider “not only where we get our training data but where we get the labels that will function in the system as a stand-in for ground truth.” Right, as I’ve written before, data reflects social biases, and the labels we use to classify people and things encode the values and beliefs of the labeler. Christian also takes the reader inside the decisions and assumptions developers make — for example, that the situations the model encounters in the real world will resemble, on average, what it encountered in training. Or that “the model itself will not change the reality it’s modeling,” but as Christian rightly notes, “In almost all cases, this is false.”
Furthermore, Christian warns that embracing AI inculcates problematic, predictive thinking, predicated on the idea that we can model the world. Christian writes,
“We are in danger of losing control of the world not to AI or to machines as such but to models. To formal, often numerical specifications for what exists and for what we want.”
I agree! It would be a big problem if we started to assume that the map actually is the territory or if we let technical systems, as Christian puts it, “enforce the limits of their own understanding.” Substituting a model of the world for the real world and all of its complexity gets closer to my concern. But Christian is ultimately telling a story of progress — of how AI researchers are making small steps forward in the march toward ‘alignment,’ as if it might just be around the corner. I don’t think that it is.
I was a civil servant in the federal government for eight years. I co-founded and ultimately led the Center for Digital Development at USAID. Two mindsets prevalent in international development at the time made this work extremely hard. The first is the ‘tech for good’ mindset I’ve written about before that “leads to the definition of a problem that requires a technology solution. It becomes a trojan horse for scaling the technology, and the interests and beliefs of its creators.” This is doubly seductive when we create organizational constructs that consider technology a vertical, and prefix team names with the word ‘digital’. But I digress.
The second mindset believes that systems are ordered wherein, as Dave Snowden, founder of the Cynefin Company, put it “there are underlying relationships between cause and effect in human interactions and markets, which are capable of discovery and empirical verification.” In other words, if I do X, Y will occur, and we can verify that X caused Y — and so, the future is only a neat theory of change away.
At USAID, something called ‘the logical framework’ guided the design of every program, and it nurtured the belief that systems are ordered. In these frameworks, we would detail how inputs lead to outputs, and then how outputs would collectively achieve the program’s purpose. Essentially, we thought it was right to assume that we could align our interventions to specific outputs.
In actual fact, many systems are unordered so we have to adjust our assumptions and decision-making processes accordingly. In the Cynefin Framework (see below), Snowden divides the world into ordered and unordered systems, where ordered systems contain:
Clear systems: this is the land of best practice and standard operating procedures. Everyone agrees on the right answer, so all you have to do once you realize that you’re in this context is categorize the problem and respond.
Complicated systems: this is the land of good practice. There are multiple legitimate responses but not everyone agrees what to do. What’s needed are experts to analyze the context, determine which response makes the most sense, and then respond accordingly.
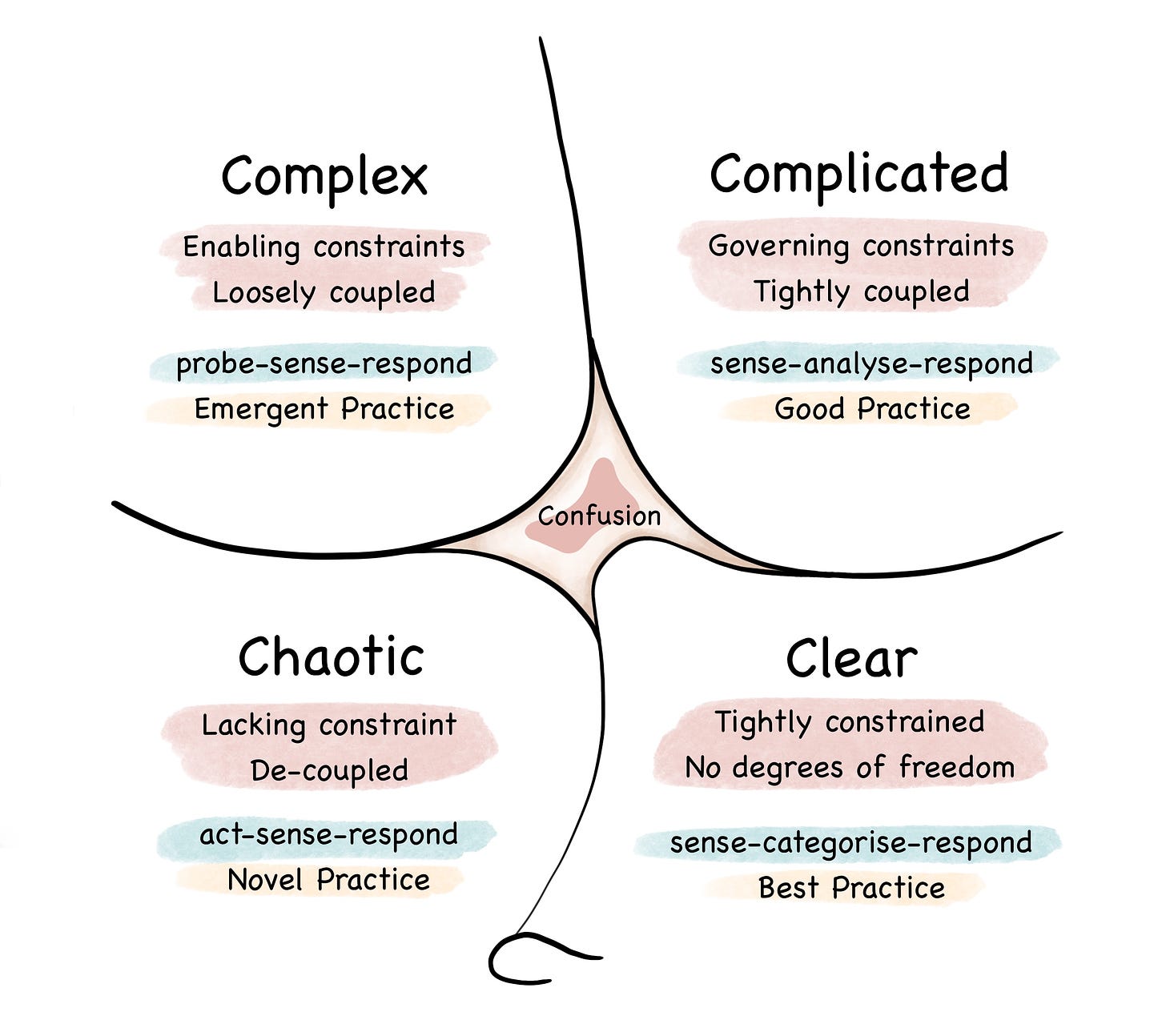
The assumption that if we do x, y will occur only holds up in contexts that are clear or complicated. But in unordered systems, which are either complex or chaotic, ‘cause and effect’ just doesn’t apply. You don’t know what to do until you act because you can’t know for sure how the system is likely to respond. So you probe it, or run “safe to fail” experiments, as Snowden calls them. As you test novel ideas, possible solutions start to reveal themselves. It’s also in complex, unordered systems wherein you need multiple, diverse perspectives on the problem — because again, it’s not clear what to do.
Unordered systems do not call for methods of best practice or even good practice, but hypotheses testing and collaboration across differences. It’s also through testing these hypotheses that the system itself changes — “agents modify the system” and “practice,” as Snowden puts it, “is emergent.” So what’s needed in an unordered system is an ongoing process of collective sense-making that draws on a diversity of perspectives.
This view of the world aligns (pun intended!) with my modest rant on ‘first principles thinking’ last month — that we need to “relax our assumption that facts are stable — they are contingent” and “relax our assumptions about what’s knowable, embedding more uncertainty and humility into our technology development, decision-making, and policymaking.” There is a prevalent idea in the tech sector that we can make systems do what we want them to do — and therefore that the alignment problem is something that can be ‘solved’. Maybe you can have influence over an ordered system. But an unordered system? Good luck!
So what to do about this?
With AI, a current goal seems to be to get it to interact with the world on a meaningful, impactful level. But if we want that to happen, we need to understand what kind of systems make up ‘the world’. They are likely systems that are much more complex than we think.
Not knowing what system you’re in is a big problem. In the Cynefin framework, this is the quadrant in the middle of the diagram above labeled ‘confusion.’ According to Snowden, this is when we start trying to make sense of the system per our personal preferences and epistemologies. For example, those in a highly bureaucratic context might see the problem as a failure of process. Others might instead refract the problem through their own political or ideological beliefs. This leads to increasing fragmentation and fracturing of our shared reality. Not great.
Moreover, when we automatically assume that we are in an ordered system — when we’re not — we play a dangerous game: we start to behave as if we just know what to do; that all we need are experts making analytical, reasoned decisions. But if the solution only ever emerges from collaborative experiments, then we’re going about it all wrong. Along the way, we start to lose trust in experts who are supposed to know the answer, when maybe we should have assumed more uncertainty and humility from the jump. Ironically, the path to progress starts by letting go of the assumption that ‘alignment’ is a problem we can solve, and accept that it has been a myth all along.
As always, thank you to Georgia Iacovou, my editor.